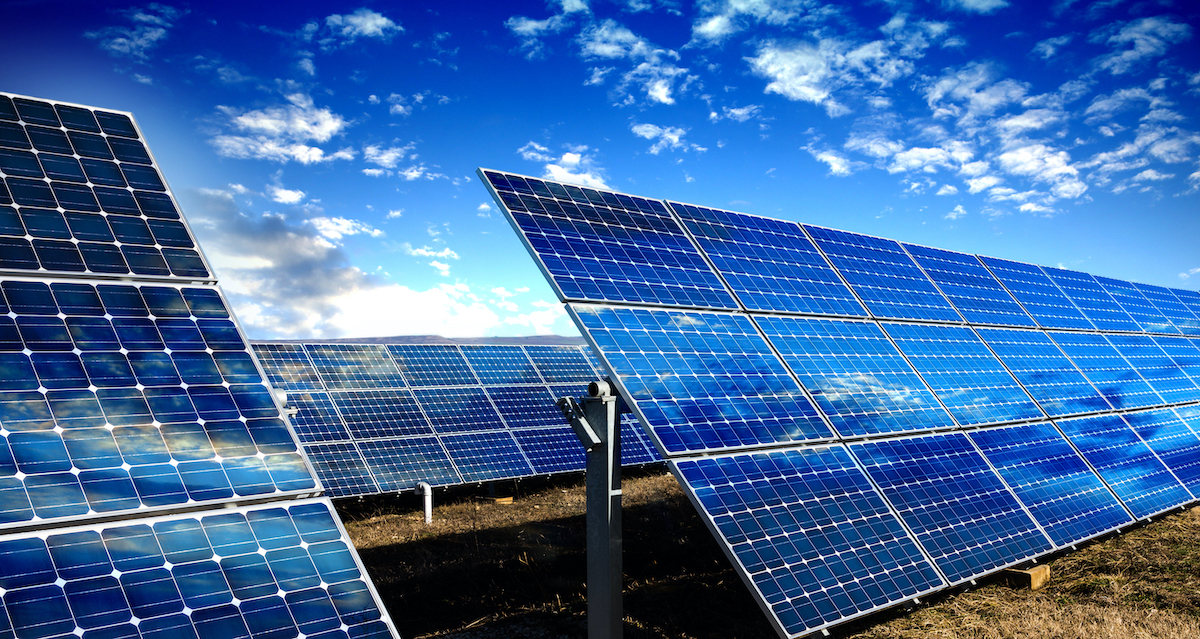
Solar PV Power Forecasting
The global need and demand for renewable energy has been rising consistently, for the first time, in 2020 renewables generated more electricity than fossil fuels across the EU, spurred on by new solar and wind power projects. With consumption growing at an average annual rate of 13.7% over the past decade, renewables were the only category of energy that grew globally at a rate in the double digits. The International Renewable Energy Agency reported that global renewable generation capacity was at 2,537 GW at the end of 2019. The rise of renewable energy sources is here to stay as the benefits are undeniable: they don’t produce greenhouse gases or pollution and are abundant in nature.
As countries and companies around the world take hard-hitting action on climate change by targeting net-zero emissions, renewable energies are becoming increasingly competitive with their costs falling and fossil fuel prices generally rising. Renewables will overtake coal to become the largest source of electricity generation worldwide in 2025, but there are a number of challenges and choices to be made when it comes to renewables. One key risk for renewable energy is political policy around incentives and support, for example, in China wind and solar PV subsidies and offshore wind support expire this year leaving uncertainty in the air.
Solar’s rapid expansion
Renewables grow rapidly in all our scenarios, with solar at the centre of this new constellation of electricity generation technologies.
Solar energy is harnessed from the sun’s rays and can be converted via silicon solar panels which is described as "photovoltaic" and generates direct current (DC) electricity directly from sunlight. It can be converted to alternating current (AC) electricity through an inverter before being directly fed into an electrical grid. A solar PV plant contains a large number of panels, configured to harvest the most light energy possible from the sun. There are several other solar power plant types that utilise different techniques to harness the power of the sun, such as solar thermal plants.
Solar power consumption is growing at around twice the rate of wind power, and is set to overtake as the leading source of renewable powe this decade. The reason for solar’s popularity is that it is the cleanest renewable energy and its source, the sun, is nearly limitless and is accessible anywhere on earth.
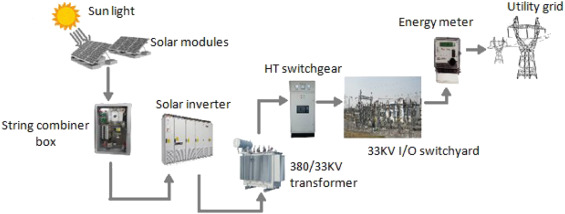
The rate at which solar panels can generate electricity varies depending on how much direct sunlight there is and the quality, size, number and location of panels being used. Solar power is therefore a variable renewable energy (VRE) and provides intermittent power. Locations with higher solar insolation will inevitably yield higher electricity production.
Solar PV plant challenges and opportunities
The integration of solar PV into power grids is challenging due to the dependency on meteorological conditions and the unpredictability of the weather. Forecasting power output is incredibly important as it can support grid operators with planning and scheduling in order to meet market demands. They’ll know whether or not they need to purchase from other plants, such as fossil fuel plants. Solar power plant owners benefit from forecasting as they have more certainty over what will be generated and can meet regulatory requirements which are present across many countries and ask that forecasts are submitted.
Economically, accurate forecasts have a major impact for solar plants and their operations. Income is associated with the electrical output and the rate at which electricity is then purchased, therefore, knowing the output is key for financial planning and success. The variety of atmospheric parameters including temperature, cloud quantity and dust make accurate PV power predictions a difficult task.
Case Study: Forecasting solar PV with a hybrid approach
At Equinox, we teamed up with a market leading oil and gas company in Central Europe who have pledged to shift their focus towards renewable and green solutions by 2030. We created a protype forecasting solution that enabled them to forecast electricity production on a daily basis for three medium-sized solar PV plants that they were running. Solar forecasts are used by our customer to meet government requirements for plant operators to submit the forecast for the grid operator, as well as to enable better day-to-day business planning and operations.
There are many methods and approaches to forecasting so the first step was to choose the most accurate form from the commonly used approaches.
These approaches typically include:
- Physical model (based on numerical weather prediction or satellite images)
- AI and machine learning
- Time series models
- Probabilistic models
- Regressive models
- A hybrid approach
AI, machine learning and time series forecasting
The chosen approach to forecasting was to use a blend of AI, machine learning and time series forecasting to forecast the weather and power output of the plants. We utilised historic data of the actual electricity generated by the plants and weather forecasts in order to generate the production forecast.
First, we tested the accuracy of the weather forecasts by periodically saving the forecasts and then comparing them with what actually occurred at the end of the day for the plants’ location. We could then see which forecasts were performing well and we were able to select the best performing weather forecast from here.
Once the weather forecast was reliable, we could then look into the production forecasting solution. We evaluated several existing solutions based on statistical and machine learning approaches, including neural networks (Tensorflow, LSTM), regression based models and random forests. In the end, we decided to use a blend of these techniques with ensemble methods.
The result encompassed several versions of electricity production forecasts:
- Day-ahead: at T (today) at 10AM produces a T+1 (tomorrow) forecast (00AM to 11:59pm)
- Intra-day: on the same day, produces a refines forecast for several hours ahead
- Long-term look ahead, for capacity planning and as an input for supply & demand
Constant evaluation and improving accuracy
The solution was designed to constantly evaluate the accuracy of its forecasts. For each solar plant, different forecasts are generated and the results back tested. The output of these tests is then incorporated into model calibration to ensure ever improving accuracy for the solar power forecasts.
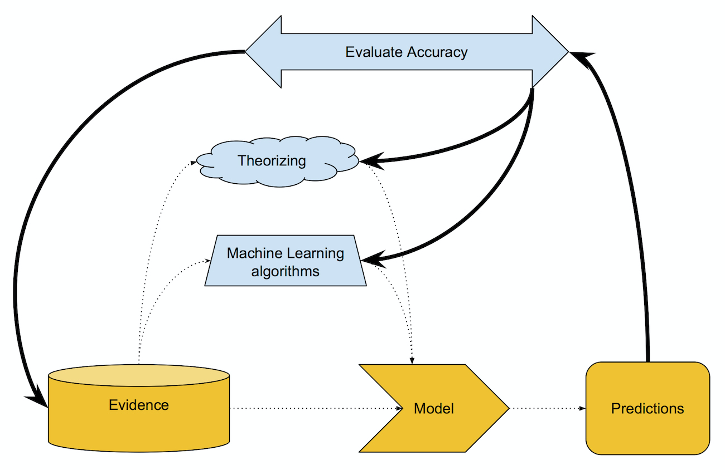
From an operations point of view, users of the forecasting tool have access to a web-based portal where they can set up and configure solar plants, check their status, schedule maintenance events and see a real-time view of solar power production and forecasts. As an enterprise solution, it has been built with stringent security controls including secure user authentication and role-based authorisation, audit logs and constant monitoring of processes.
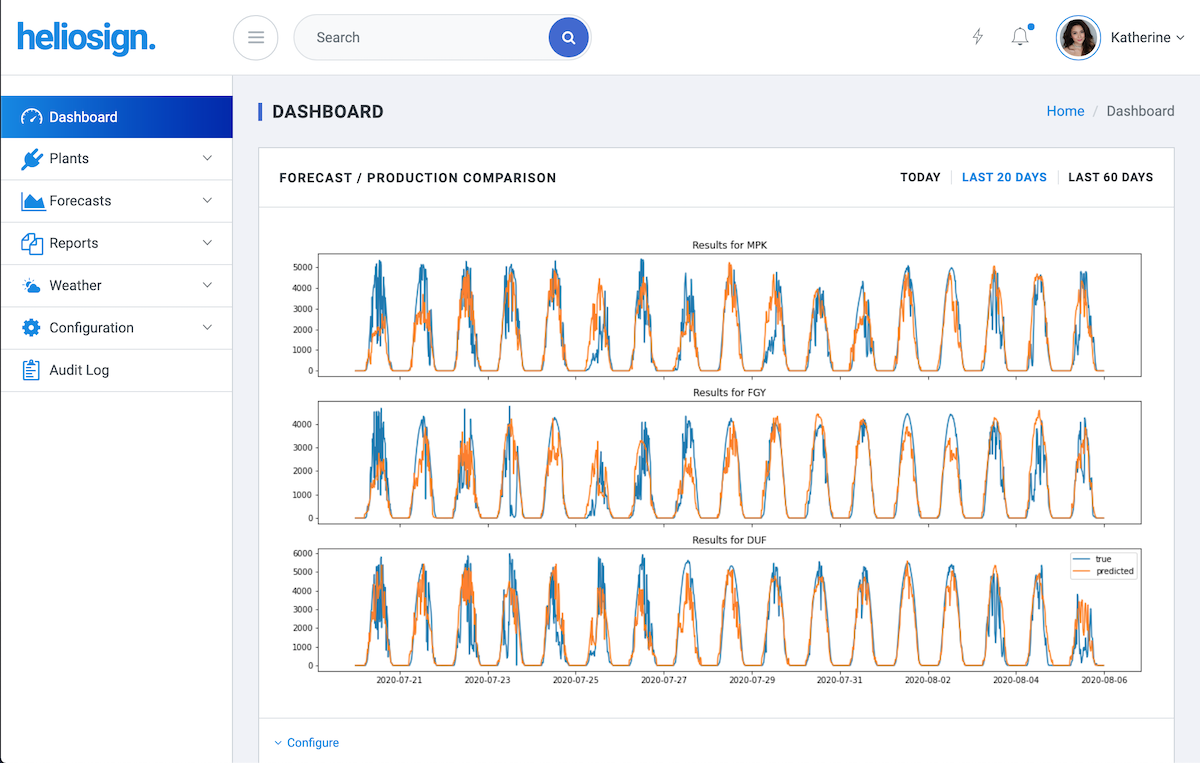
Future improvements and use cases
Accurate solar power forecasting is a critical element in the success of integrating solar PV into the grid.
It addresses the needs of different operation and control activities, including grid regulation, power scheduling, and unit commitment in both the distribution and transmission grids.
Future improvements to the hybrid approach to solar PV forecasting could involve expanding to probabilistic weather forecasting which uses predictive probability distribution over future quantities or events of interest. There are several studies on probabilistic solar power forecasting that show its potential.
For plant owners and operators a virtual plant can be a useful solution, to help forecast and plan production capabilities, negotiate PPA to secure future energy sales, also to optimise size of energy storages.
Solar power forecasting is a key tool for energy traders as an accurate forecast could mean the ability to increase profits through better decision making. It can be used to track power forecasts across a portfolio of plants or at a national level, providing optimisation of trading positions and an early understanding of the market.
To learn more about solar power forecasting and our solutions, get in touch.