Customer Success Story
Mobile-Only Bank
Financial services companies generate and compile exabytes of data a year, including structured data such as customer demographics and transaction history and also unstructured data such as customer behaviour on websites and social media.
With the recent trends of surging fintech companies and increased demand for digitalisation and automation throughout the finance sector a need for a better way of handling data and producing outputs from it.
Starting with the financial crisis in 2009, financial institutions have increased their focus on customer risk management. Around the globe, financial organisations continue to lay down rules for credit risk and liquidity ratio levels, including regulatory acts such as AML, Basel III and FATCA that increase the amount of customer data available for analysis.
Also, financial institutions now have to filter through much more data to identify fraud. Analysing traditional customer data is not enough as most customer interactions now occur through the Web, mobile apps and social media.
To gain a competitive edge, financial services companies need to leverage big data to better comply with regulations, detect and prevent fraud, determine customer behaviour, increase sales, develop data-driven products and much more.
Challenges
In the finance sector, every type of company has large datasets. These can be various, depending on the company’s focus, whether it is a retail, investment, wealth management or insurance oriented. These data, for example, transactional data, customer data, market and reference data, are often scattered through the company’s databases and other unstructured data storages. Often these datasets belong to a particular group inside the organisation. Usually, unfortunately, there is no connection between them, might even storing the same data in various formats in multiple places. Under these circumstances, the delivery time is slower and capabilities of technology departments which makes it hard for the business to experiment with new ideas, strategies, frequently causing a situation that by the time the prototype or the first iteration of the solution is delivered the business has already moved on.
Companies use enterprise data warehouses (EDW), which are critical for enabling operational reports for businesses – but as the size and complexity of the data to be analysed increases, you’ll eventually hit the limits of traditional data warehouses.
You’ll know it when your processing times take too long to meet business needs, your costs get out of control, or you struggle to process and analyse new data types. For both IT executives and key stakeholders responsible for analytics, business intelligence and enterprise data, this is a severe problem. Today’s business decision makers can’t afford delays in insights anymore.
The solution is to offload the most challenging data management and analytics activities to new technologies and management approaches designed to handle them. For example, do you need to cut the costs of data preparation and cleansing? Reduce time to insight by offloading the most time-consuming analytical tasks? Support a variety of new data types, especially unstructured data? Or better manage rapidly growing log, sensor and other unstructured data?
Traditional EDWs were never designed to solve these types of challenges. First, they make it prohibitively expensive to manage the ever-increasing volumes of transaction and interaction, mobile data, website clickstream data, ad click-through, log data, sensor data, and unstructured machine data. At second, they’re slow to produce analytics from unstructured data because they don’t support it. This forces technicians to give this data structure before analysing it manually.
Solution
The good news is, big data analytics solutions that run on Hadoop can solve these challenges. Big data analytics solutions running on Hadoop make it easy to overcome these challenges because they allow you to cost-effectively scale to any volume of data and store and analyse all data types together – both structured and unstructured. You can also use them to extract structured data from your EDW into Hadoop for cheaper storage and then send back into EDW for analytics. All data can be analysed as is, eliminating costly data preparation activities. At the same time, big data analytics is so powerful because it enables you to combine, integrate and quickly analyse all of your data at once – regardless of source, type, size, or format – to generate the insights your business needs. In addition, you can parse, clean, profile, match, enrich, aggregate, and normalise data, as well as manage ETL workloads and generate master data.
We provide a one-stop solution for getting all of your Web, advertising, mobile, social media, transaction, marketing automation, and CRM data into Hadoop; enriching it with third-party data; analysing your data; and visualising results using wizard-led data integration, point-and-click analytics functions, and drag-and-drop visualisations.
Our broad set of data connectors and analytic functions make it easy to:
- Rapidly combine and enrich existing datasets with third-party, customer, and other data
- Perform ad-hoc analytics to test what-if scenarios
- Better understand your customer interactions and identify the most effective campaigns
- Identify the behaviours of customers at risk of churn
As a result, you can answer questions like:
- What’s happening across the customer journey?
- Which campaign combinations accelerate revenue?
- Which offers drive customer loyalty?
- What credit card behaviour signals potential fraud?
- How can we assess customer risk before extending credit?
- How can you create more targeted campaigns?
The following case study outlines how one of our client, a progressive financial services company using workflow-based automation and big data for a competitive advantage.
Client
The client for this project was a neobank, a fintech company with the ambition to become a fully functional and operational bank, building their company from the ground up, while only having a mobile app as a facade for its customers. This new business model appeals to many customers, and several companies are offering such services. Their offering is mainly built around low operational costs which allows them to give their customers low service rates for current accounts, foreign exchange transactions (spending abroad), personalised saving incentives and lending offerings.
The IT implementation was a greenfield project, meaning that all the components either had to be developed or a vendor solution had to be integrated. We were involved in solutions for fraud, finance and operations on the back-end.
Finance
The company used several external SaaS accounting solutions and was using data from internal data sources as well, like a system of record and general ledger. The project was to create real-time data pipelines for their systems and to ingest the data into a data lake. Once the data was in the lake, it could be quickly joined together, and reporting was made easy using several data sources.
This solution enabled the company to have an overarching profit and loss report overseeing all entities and datasets.
Operations
Customer and internal activities generate a massive amount of transactions every day. These transaction records are for originating in various source systems and for different purposes. Some represent a transaction made by a customer (i.e. top-up or payment), some belong to the bank’s internal activities such as moving funds between external bank accounts or making foreign exchange transactions. All these transactions have a lifecycle, meaning that after origination, they need to be cleared and settled. To achieve that transactions are processed repeatedly, usually every day. This is often called as end of day batch processing. One daily run consists of several workflows, and each workflow is formed of multiple steps.
Our solution was a tool, which allowed them to visually create these workflows, define dependencies between them and to run and monitor them. Having an end of day processing in place enabled the bank to automate large chunks of manual labour for daily activities around FX transactions, payment settlement and real-time reconciliation.
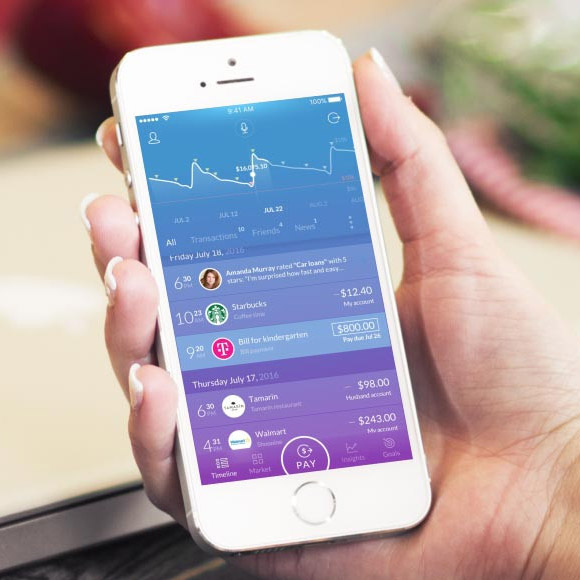
12
Project Months
Data Strategy, Financial Data, Fraud Detection, Back Office
Initiatives
Amazon Web Services (AWS), Microservices
Java, PostgreSQL
Key Technologies